Low Auto-Correlation Binary Sequences Explored using Warning Propagation
Abstract
The search for binary sequences with low auto-correlations (LABS) is a computationally hard discrete combinatorial optimization problem. We explore two physically inspired algorithms to explore the low energy space of this model. The greedy, T = 0, Monte Carlo (MC) method gets trapped in the exponentially many 1-Spin-Flip stable configurations, that are typically low in energy, but still far from the global optimum. The more elaborated Warning Propagation (WP) algorithm also gets trapped in local minima. However, these local minima, are more stable to spin flips than the ones obtained by the greedy MC. We also compare the behavior of both algorithms in randomized versions of LABS, showing that the low energy space of the 4-Spin model is easier to explore than the one of LABS.
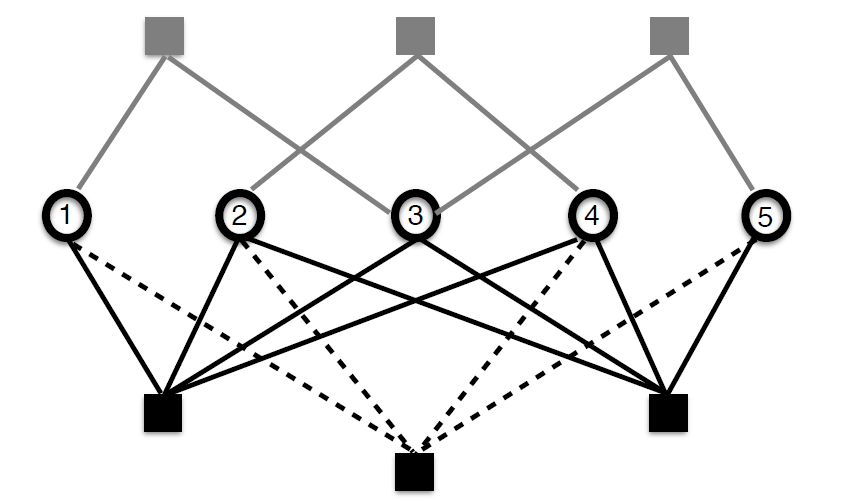

This work is licensed under a Creative Commons Attribution-NonCommercial 4.0 International License.
This work is licensed under the Creative Commons Attribution-NonCommercial 4.0 International (CC BY-NC 4.0) license.